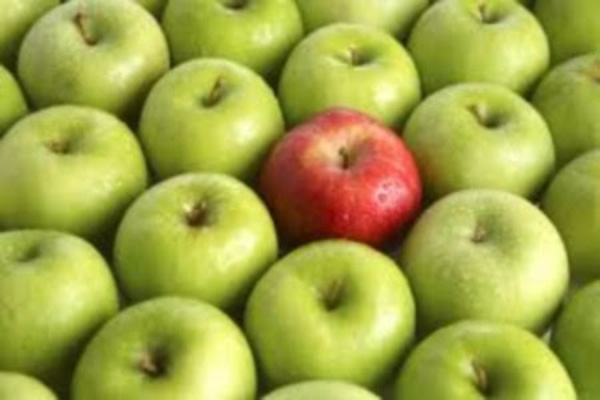
Explore Our Solutions
We help companies build better planning and forecasting capabilities
We help companies build better planning and forecasting capabilities
Planning & forecasting services by widely experienced analysts using award-winning forecasting systemÂ
Financial Panning and Analysis Services by experienced Financial analysts using state of art system.
Implement state-of-the-art business intelligence platform and take your planning process to the next level
Find opportunities and hire best planning talent by using our expertise and reach
We provide planning education and training to planning function as well as planning professionals and students to improve their skill sets
We help companies build better planning and forecasting capabilities
Planning & forecasting services by widely experienced analysts using award-winning forecasting systemÂ
Financial Panning and Analysis Services by experienced Financial analysts using state of art system.
Implement state-of-the-art business intelligence platform and take your planning process to the next level
Find opportunities and hire best planning talent by using our expertise and reach
We provide planning education and training to planning function as well as planning professionals and students to improve their skill sets
Regression is one of the highly spoken about quantitiative methods in forecasting. It is a statistical method of identifying relationship between two or more variables (one dependent variable and others independent variables). If these two variables have a strong relationship then we leverage this relationship to use the knowledge of the independent variable to predict the dependent variable. For instance if we know that historically the sale of a cola drink has a strong relationship with the temperature then we can use the temperature data to predict the sale of the cola drink.
We first identify the nature of relationship between the dependent variable and the independent variable. After identifying the relationship, we determine the effect of these independent variable on the dependent variable. Take another example, if we want to determine the next month sales and we know that the sale is highly affected by the price changes. So, the next month’s sale is dependent on the price. This makes the sale as dependent variable and price an independent variable. In this scenario when the price goes up the sales go down and vice versa. So, there is a strong relationship between the two. We can now leverage this relationship to estimate how much sales would happen when there is a change in price for that product.
There can be multiple variable affecting the dependent variable. For example, sale can be affected by seasonal effects, direct and indirect competition, purchasing power of consumer etc. These variables should not be interdependent as then they would not be truly independent variable. As discussed, you would need to identify the relationship between the independent variable and independent variable.
How to determine the relationship between two variables?
1.Visual Inspection on scatter plot: When you plot the two variables on the scatter plot then you can easily see what kind of relationship is there. For example, when the price increases the sale reduces this shows that there is a linear direct relationship between them. If the increase in price also increased the sale then there is linear direct positive relationship between the two variables.
Image Source: Google
2.Statistical analysis : The degree of relationship can be statistically estimated using correlation factor. Correlation value lies between -1 to +1, +1 indicating strong correlation and 0 indicating no correlation while -1 indicates strong negative correlation.
After identifying a strong relationship between the two variable you can predict the outcome of the dependent variable if you know the independent variable value.
Limitations
While regression is one of the exciting techniques to use, one needs to be cautious before using this technique. Unfortunately due to the nature of the technique it is usually less accurate. The reason being that you need to depend on the accuracy of the independent variable to get an accurate forecast. So there needs to accurate forecast available for factors such as weather, price, consumer power etc before we can leverage it for forecasting. This dependency makes this technique a bit difficult to deploy.
Secondly, you cannot always apply regression technique upto the part number. For instance you cannot really establish a strong correlation of temperature with every variant of drink but perhaps only to cola as product category. The individual variants demand may depend on its flavor or other consumer preferences. This makes this technique more suitable for strategic planning or marketing purposes rather than for supply chain and logistics purpose.
As a fundamental rule, you must first test with time series and then apply regression method. Ideally the regression method should be used only if it improves the results obtained from the time series methods.
Usage
Regression is commonly used to predict the impact of an event on business. Sales of products(like oil, cars) can be predicted which have strong correlation with variables (price, purchasing power, demographics etc). It can be used to predict the number of viewers who will watch a IPL which companies can use to determine how much to invest in an advertisement. In sectors like Agriculture regression is almost a given because the dependency on the weather related factors as well as the commodity pricing is very high, and usually time series methods are not reliable. However, in most other cases and specially in consumer products industry, time series methods do a better job and are deployed more commonly than regression.
Today business are diversifying and are adding new range of products or improving a new product. This make regression more challenging as each product would have different independent variable and there could be multiple variables. Thus for new products you would have to determine the variables and compute the relationship and then determine the demand of the new product. In addition to this is the list of existing products which runs into thousands. This means that a business planner not only work on the existing data and check their sanity but also determine the new variables. Hence, the regression technique should be used judicially and as an ace up the sleeve for specific scenarios where time series methods do not give desired results.